Introduction:
The dawn of Artificial Intelligence (AI) has ushered in a new era in various fields, and healthcare is no exception. This cutting-edge technology holds immense potential to transform the landscape of medical care, offering innovative solutions and enhancing patient outcomes.
Section 1: The Current State of AI in Medicine
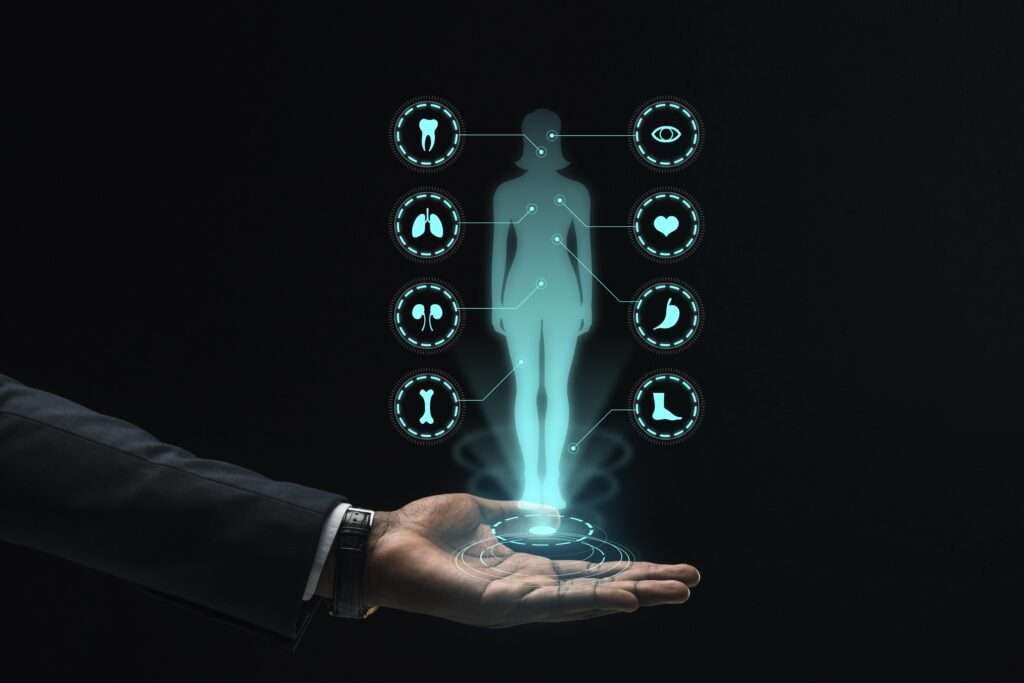
Artificial Intelligence (AI), once a figment of science fiction, now permeates the fabric of modern medicine, offering a new lens through which we can understand and enhance patient care. This section provides a snapshot of the diverse landscape of AI applications currently employed in the medical field and the profound effects these tools have on healthcare delivery.
Medicine AI Defined
The term ‘medicine AI’ refers to the application of artificial intelligence technologies, including machine learning, natural language processing, and neural networks, to solve complex problems in healthcare. It is a multifaceted domain where AI is used to process vast amounts of health data, from electronic health records to genetic information, with greater speed and accuracy than humanly possible.
Examples of AI in Healthcare
Several pioneering examples of AI in healthcare have demonstrated its vast potential. Machine learning algorithms are now adept at interpreting radiological images, spotting nuances that may escape even the trained eye, leading to earlier and more accurate diagnoses of conditions like cancer and fractures. AI-powered chatbots are providing preliminary medical advice and triaging patients, ensuring efficient use of healthcare resources. Predictive analytics is being utilized to forecast patient admissions and identify those at risk of chronic diseases, enabling preemptive care measures.
AI’s Role in Diagnostics and Treatment
AI’s analytical prowess is revolutionizing diagnostics by identifying disease patterns and predicting patient trajectories with astonishing precision. In treatment planning, AI systems analyze data from past treatments and outcomes to recommend personalized therapy regimens, considering factors that range from molecular profiles to lifestyle aspects of patients.
Patient Monitoring and AI
Continuous patient monitoring, once a resource-intensive task, is being transformed by AI. Wearable devices now monitor vital signs and alert healthcare providers to abnormalities in real time, facilitating early intervention. Similarly, AI systems in intensive care units analyze data points to detect early signs of deterioration, enabling prompt and often life-saving responses.
As AI technology becomes more sophisticated and integrated into the healthcare system, the paradigm of patient care evolves from reactive to predictive, ushering in a new era where prevention is as fundamental as the cure. The promise of AI in medicine is not in replacing human clinicians but in augmenting their capabilities, providing them with powerful tools to enhance decision-making and patient outcomes. In this light, the current state of AI in medicine is both a testament to human ingenuity and a prologue to the future of healthcare.
This section outlines the present integration of AI in medicine, its definitions, examples, and roles in diagnostics and patient monitoring, aiming to inform the reader about the real-world implications and applications of AI in healthcare.
Section 2: Breakthroughs and Innovations
The terrain of medicine is fertile ground for AI-driven breakthroughs, each innovation marking a stride towards a future where healthcare is more proactive, personalized, and accessible. This section shines a light on the pivotal AI advancements in medicine and the profound impact these technologies have on patient care.
AI-Driven Breakthroughs in Medical Diagnostics
One of the most groundbreaking achievements of AI in medicine is its ability to enhance diagnostic accuracy. For instance, deep learning algorithms have been developed to detect skin cancer by analyzing images of skin lesions with a level of precision comparable to dermatologists. Similarly, AI applications in ophthalmology can now identify diabetic retinopathy from retinal scans, providing a quick and non-invasive diagnostic tool.
Personalized Medicine and Genomics
The integration of AI with genomics has paved the way for personalized medicine, tailoring treatment to the individual’s genetic makeup. AI systems can now process and interpret the vast and complex data from human genomes to identify mutations and link them to specific diseases, leading to highly targeted therapies. This approach has notably advanced the treatment of certain cancers, where AI-guided analysis of tumor genetics can predict how well a patient will respond to specific drugs.
Case Studies: AI’s Impact on Patient Outcomes
The real-world impact of AI is best illustrated through case studies that demonstrate improved patient outcomes. For example, an AI system used in a major hospital was able to predict acute kidney injury up to 48 hours before it occurred, allowing for preventive measures that significantly reduced the progression of the condition. Another instance is the use of AI in managing heart diseases; algorithms analyzing electrocardiograms (ECGs) have been able to detect atrial fibrillation earlier than traditional methods, leading to timely intervention and better management of the condition.
Operational Efficiency and Predictive Analytics
Beyond direct patient care, AI has introduced efficiencies in hospital operations. Predictive analytics applied to patient admission data helps in managing hospital bed availability and staff allocation, leading to more efficient healthcare delivery and reduced patient wait times. AI systems are also being used to predict patient no-shows and cancellations, allowing for real-time schedule adjustments and optimizing the use of healthcare resources.
The innovations and breakthroughs in AI are not only enhancing current medical practices but are also carving out new therapeutic pathways. They exemplify AI’s potential to transform healthcare into a system that can predict illness, create personalized treatment plans, and deliver care with unprecedented precision. As these technologies continue to evolve, they promise to unlock further capabilities that will undoubtedly redefine the landscape of healthcare as we know it.
This section articulates the significant innovations AI has brought to the medical field, offering examples and case studies to illustrate how AI has tangibly improved patient outcomes and operational efficiencies in healthcare settings.
Section 3: Implementing AI in Healthcare Settings
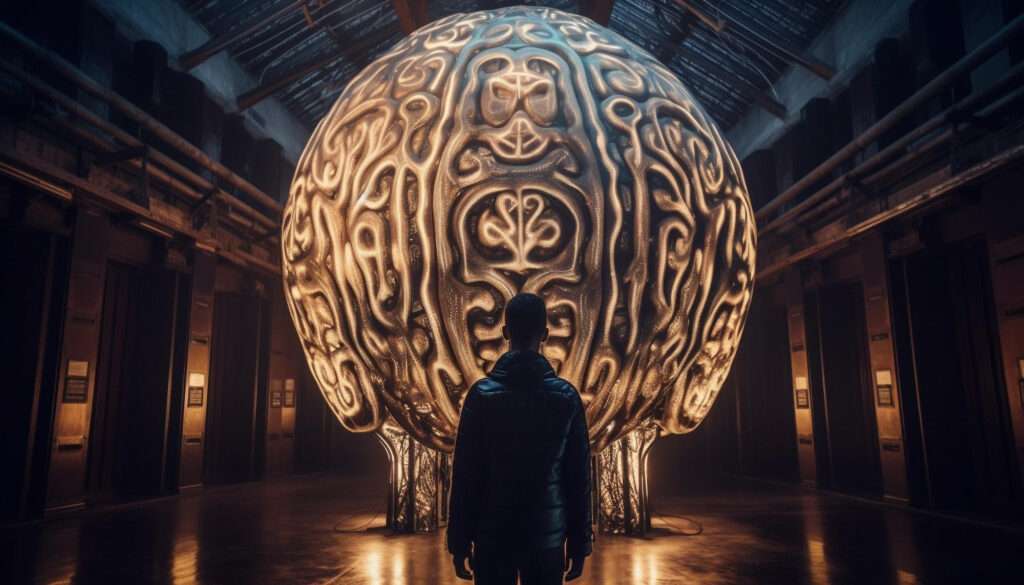
Implementing AI in healthcare settings involves integrating advanced technology into existing medical frameworks. This process requires careful planning, collaboration, and adherence to medical standards and regulations.
Strategies for AI Integration
- Assessing Needs and Goals: Identify specific areas within healthcare operations where AI can bring the most benefit, such as diagnostics, patient care, or administrative tasks.
- Infrastructure and Technology Alignment: Ensure the healthcare facility’s technological infrastructure can support AI applications. This might involve upgrading hardware, ensuring robust data storage capabilities, and establishing secure data transmission channels.
- Data Management and Governance: Develop a comprehensive data management strategy that ensures data quality, security, and compliance with regulations like HIPAA. This includes anonymizing patient data for use in AI models while maintaining its integrity.
- Collaboration with AI Vendors and Experts: Partner with AI technology providers and consultants who have experience in healthcare applications. These collaborations can aid in customizing AI solutions to meet specific healthcare needs.
- Staff Training and Change Management: Educate healthcare staff about AI tools and their benefits. Effective change management strategies can facilitate a smoother transition and integration of AI into daily operations.
Importance of AI Monitoring in Healthcare
Continuous monitoring of AI systems is critical for several reasons:
- Quality Control: Regular monitoring ensures that AI applications perform as expected and deliver accurate results.
- Adaptability and Improvement: AI models may require updates and improvements based on real-world performance and emerging healthcare trends.
- Ethical Compliance: Monitoring helps in adhering to ethical standards, ensuring AI applications do not introduce bias or harm to patients.
Steps for Healthcare Providers to Adopt AI Tools
- Pilot Testing: Before a full-scale implementation, conduct pilot tests to evaluate the AI tool’s effectiveness and identify potential challenges in a controlled environment.
- Stakeholder Engagement: Engage with all stakeholders, including clinicians, administrative staff, and patients, to gather feedback and foster a sense of ownership in the AI implementation process.
- Regulatory Compliance: Ensure all AI tools comply with healthcare regulations and standards. This includes FDA approvals for medical devices and software, where applicable.
- Performance Evaluation and Feedback Loop: Establish metrics to evaluate the performance of AI tools and create feedback mechanisms to continuously improve AI applications based on user experience and outcomes.
- Scalability and Expansion:
- Once proven effective, expand the use of AI tools from pilot areas to other parts of the healthcare organization, adjusting strategies as necessary based on earlier experiences.
Implementing AI in healthcare is a dynamic process that demands ongoing attention to technology trends, patient needs, and regulatory landscapes. By following these steps, healthcare providers can effectively adopt AI tools, enhancing patient care and operational efficiency.
Section 4: Challenges and Ethical Considerations
While the integration of Artificial Intelligence (AI) in medicine heralds a new epoch of efficiency and precision, it also introduces a spectrum of challenges and ethical considerations. This section delves into the potential pitfalls of AI deployment in healthcare and the strategies that can be employed to navigate these complexities.
Data Privacy and Security
One of the most pressing concerns is the safeguarding of patient data. AI systems require vast datasets to learn and make accurate predictions, which often include sensitive personal health information. The risk of data breaches and unauthorized access to this information poses significant privacy concerns. Robust cybersecurity measures, clear data governance policies, and stringent compliance with regulations like the General Data Protection Regulation (GDPR) and Health Insurance Portability and Accountability Act (HIPAA) are essential to protect patient information.
Bias and Fairness
AI is only as unbiased as the data it is trained on. If the underlying data contains historical biases or lacks diversity, AI systems can perpetuate or even exacerbate these issues, leading to unfair treatment recommendations or diagnostic errors. To combat this, it is crucial to use balanced datasets that are representative of the entire population and to design AI algorithms that are transparent and can be audited for bias.
Informed Consent and Transparency
Patients must be informed about how their data is being used and the role AI plays in their care. This includes understanding the potential risks and benefits of AI-driven medical decisions. Transparency in AI-driven processes and outcomes, along with ensuring that patients provide informed consent, are fundamental ethical obligations.
Accountability and Liability
Determining accountability for AI decisions can be challenging. If an AI system makes a recommendation that leads to a medical error, it is not always clear who — the healthcare provider, the AI developer, or another party — should be held responsible. A framework for legal and professional accountability that addresses these unique scenarios is needed.
Mitigating the Challenges
To mitigate these challenges, continuous oversight and adaptive regulatory frameworks are required. This includes setting standards for AI model development, validation, and continuous monitoring once deployed. Ethics committees specifically focused on AI in healthcare can provide guidance and oversight. Ongoing training for healthcare professionals on the capabilities and limitations of AI will also be essential.
Moreover, interdisciplinary collaboration between technologists, clinicians, ethicists, and legal experts is vital to ensure that AI is developed and implemented in ways that respect patient rights and promote the best clinical outcomes. It is through these concerted efforts that the healthcare sector can fully harness the potential of AI while maintaining the trust and well-being of patients.
This section provides a critical analysis of the challenges associated with the implementation of AI in medicine, emphasizing the importance of ethical considerations and suggesting ways to address these issues to ensure that the benefits of AI can be realized without compromising patient safety and privacy.
Conclusion:
AI in medicine is not just a futuristic concept; it’s a present-day reality with the potential to revolutionize healthcare. As AI continues to evolve, its impact on the future of medicine is undeniable, promising a new horizon of personalized and efficient patient care.